Post by BCcampus Student Research Fellow Marta Samokishyn
Why Talk About Algorithmic Literacy?
Today we encounter algorithms almost everywhere: when you scroll through social media, algorithms determine what you see; when you apply for a loan, algorithms determine if you are approved and for how much; when you are given an insurance quote, algorithms decide how much you will pay; when you search for scholarly sources, algorithms offer their recommendations. They are embedded into our current socioeconomic structures and other aspects of everyday life, often including academic work.
According to Kitchin (2017), “algorithmic power” to make many significant decisions impacts and “reshape[s] how social and economic systems work” (p. 16). Algorithmic power can be highly problematic due to its systemic biases about race, gender, income, household location, and other factors, as demonstrated in decisions of algorithms, such as which population groups see certain information online and which do not (Kitchin, 2017). For example, researchers from Carnegie Mellon University found Google ads were gender-biased in offering higher-paying jobs to men and not to women (Datta et al., 2015). Another example of algorithmic bias is racial bias of crime-prediction software, which targets Black and Latino neighbourhoods (Sankin et al., 2021). Social media offers countless examples of algorithmic biases, including the homogeneity of Facebook’s news feed and targeting content for specific population groups (Morris & Mattu, n.d.). See an excellent example on The Markup.
Algorithmic Literacy in the Context of Other Literacies
The above urges us to address the issue of algorithmic literacy and its role in both academia and beyond. What does it mean to be algorithm-literate? For this project, I am adopting the following definition of algorithmic literacy:
Algorithm literacy can … be defined as being aware of the use of algorithms in online applications, platforms, and services, knowing how algorithms work, being able to critically evaluate algorithmic decision-making as well as having the skills to cope with or even influence algorithmic operations. (Dogruel et al., 2022, p. 118)
Dogruel et al.’s definition echoes major information-literacy concepts, such as searching and evaluating information sources. However, it also broadens them by incorporating different forms of literacies. Many authors agree algorithmic literacy needs to be re-envisioned as part of metaliteracy, as different types of literacies, such as digital and cyber literacies, media literacy, and visual literacy, have become an integral part of information-literacy education (Gersch et al., 2016; Mackey & Jacobson, 2014).
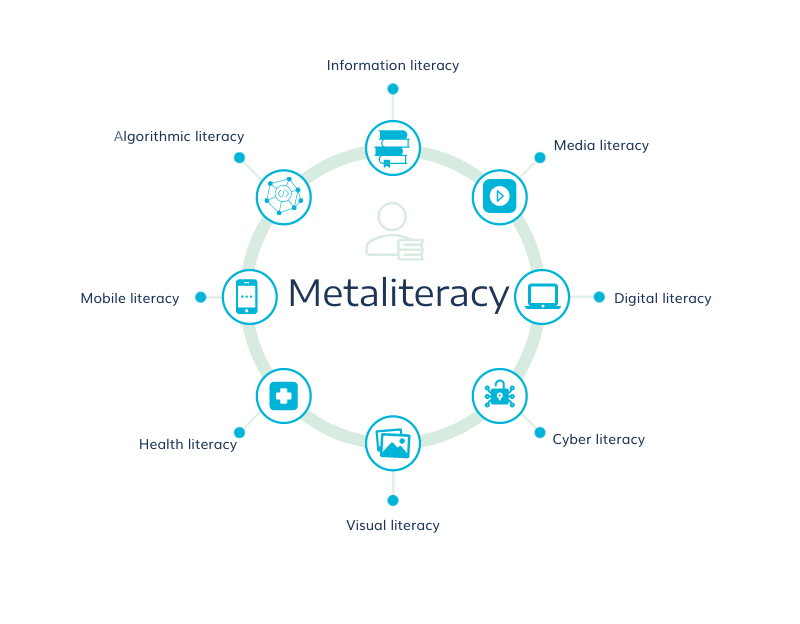
According to Mackey and Jacobson (2014), “Metaliteracy expands the scope of traditional information skills … to include the collaborative production and sharing of information in participatory digital environments” (p.1). The vision of algorithmic literacy as metaliteracy further expands our understanding of how we think about algorithms and whose role it is to talk about algorithmic literacy in higher education. Several authors state that algorithmic literacy should be included in information-literacy education in academic libraries as a way to bring critical awareness to how algorithm-ranking works and how algorithmic bias impacts social and economic structures today (see Bakke, 2020; Eaton, 2022; Ridley & Pawlick-Potts, 2021; Shin et al., 2021).
This project will explore algorithmic literacy in the context of information-literacy interventions in higher education and invite learners to adopt a critical approach to algorithms as they navigate and live in a “black box society” (Pasquale, 2016).
This research is supported by the BCcampus Research Fellows Program, which provides B.C. post-secondary educators and students with funding to conduct small-scale research on teaching and learning as well as explore evidence-based teaching practices that focus on student success and learning.
Did you know? Marta is presenting on this topic at ETUG on November 4, 2022. To learn more visit the ETUG Fall 2022 event page.
Relevant links:
- 2022–2023 Student Research Fellows
- 2022–2023 Student Research Fellow: Marta Samokishyn
- FLO Lab: Staying Current with Essential Digital Literacy
References:
Bakke, A. (2020). Everyday Googling: Results of an observational study and applications for teaching algorithmic literacy. Computers and Composition, 57, 102577. https://doi.org/10.1016/j.compcom.2020.102577
Datta, A., Tschantz, M. C., & Datta, A. (2015). Automated experiments on ad privacy settings: A tale of opacity, choice, and discrimination (arXiv:1408.6491). arXiv. https://doi.org/10.48550/arXiv.1408.6491
Dogruel, L., Masur, P., & Joeckel, S. (2022). Development and validation of an algorithm literacy scale for internet users. Communication Methods and Measures, 16(2), 115–133. https://doi.org/10.1080/19312458.2021.1968361
Eaton, C. D. (2022). Teaching machine learning in the context of critical quantitative information literacy. Proceedings of Marching Learning Research, 170, 51–56. https://proceedings.mlr.press/v170/eaton22a.html
Gersch, B., Lampner, W., & Turner, D. (2016). Collaborative metaliteracy: Putting the new Information Literacy Framework into (digital) practice. Journal of Library & Information Services in Distance Learning, 10(3–4), 199–214.https://doi.org/10.1080/1533290X.2016.1206788
Kitchin, R. (2017). Thinking critically about and researching algorithms. Information, Communication & Society, 20(1), 14–29. https://doi.org/10.1080/1369118X.2016.1154087
Mackey, T. P., & Jacobson, T. E. (2014). Metaliteracy: reinventing information literacy to empower learners. American Library Association.
Morris, S. & Mattu, S. (n.d.). Split screen: How different are Americans’ Facebook feeds? The Markup. https://themarkup.org/citizen-browser/2021/03/11/split-screen
Pasquale, F. (2016). The black box society: The secret algorithms that control money and information. Harvard University Press.
Ridley, M., & Pawlick-Potts, D. (2021). Algorithmic literacy and the role for libraries. Information Technology and Libraries, 40(2), Article 2. https://doi.org/10.6017/ital.v40i2.12963
Sankin, A., Mehrota, D., Mattu S., & Gilbertson, A. (2021, December 2). Crime prediction software promised to be free of biases. New data shows it perpetuates them. The Markup. https://themarkup.org/prediction-bias/2021/12/02/crime-prediction-software-promised-to-be-free-of-biases-new-data-shows-it-perpetuates-them
Shin, D., Rasul, A., & Fotiadis, A. (2021). Why am I seeing this? Deconstructing algorithm literacy through the lens of users. Internet Research, 32(4), 1214–1234. https://doi.org/10.1108/INTR-02-2021-0087
© 2022 Marta Samokishyn released under a CC BY license
The featured image for this post (viewable in the BCcampus News section at the bottom of our homepage) is by Christina Morillo